POSTS
Method development
Single molecule tracking data usually contains large amounts of information, but extracting the data from the highly fragmented trajectories, which is often the result of SPT in vivo, can be a real challenge. Traditional methods use Mean Squared Displacement and/or Cumulative Distribution Function analysis to identify parameters in presumed underlying models, but the model has to be guessed a priori and introduction of additional states does always lead to a better fit. In a paper that was recently published in Nature Methods, we describe an analytical tool based on a variational Bayesian treatment of hidden Markov models that combines the information from thousands of short single-molecule trajectories of intracellularly diffusing proteins. The method identifies the diffusion constants and state transition rates as well as the number of states in the model.
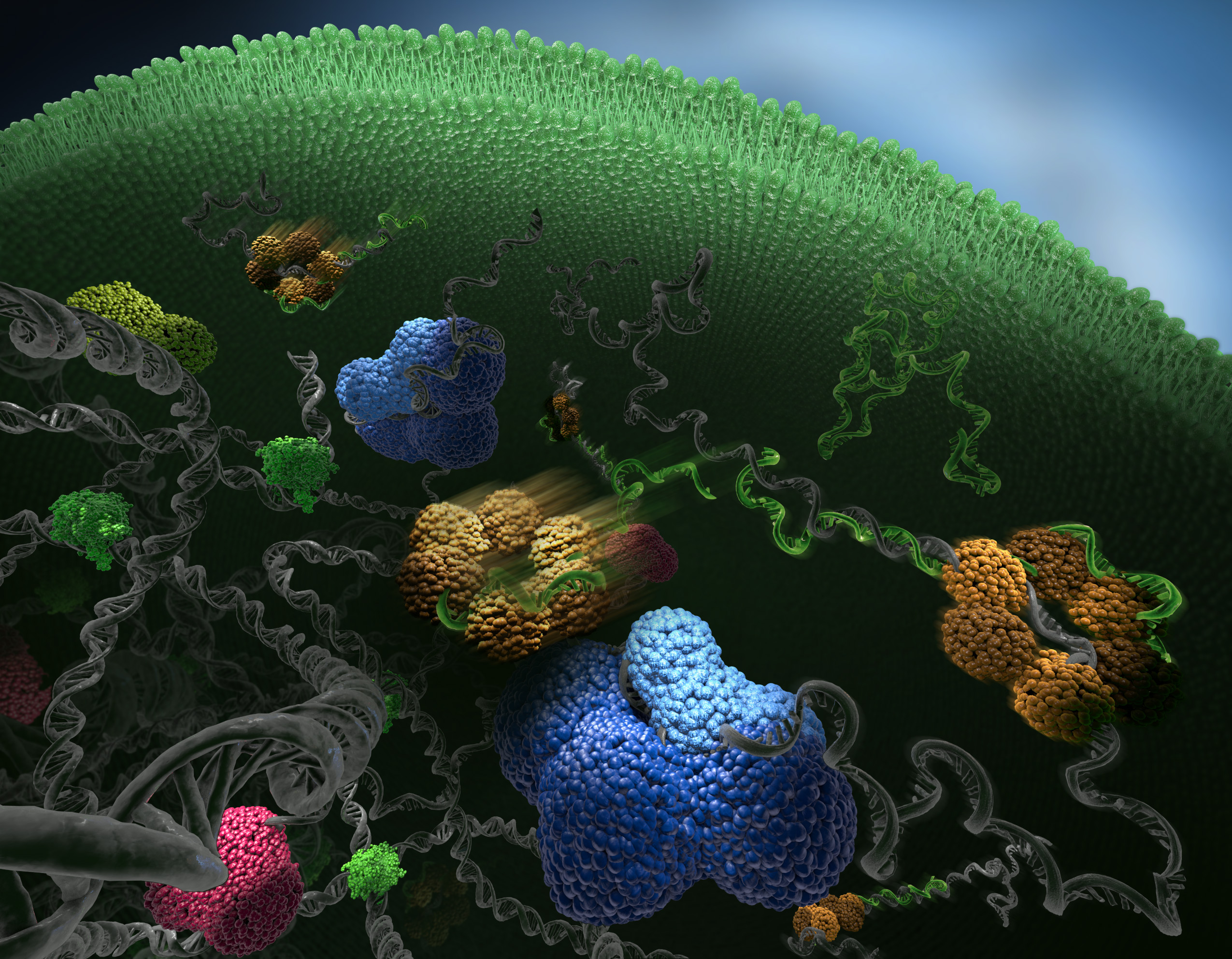
Using this method we have created an objective interaction map for Hfq, a protein that mediates interactions between small regulatory RNAs (green) and their mRNA targets (grey), see image to the right. Photoconvertable proteins were used to track single hfq molecules (yellow) and assign them to different kinetic states based on their diffusive properties. The diffusion constant of hfq depends on its state of binding. Free hfq diffuse fast, but when the molecule is bond to other molecules, e.g. mRNA, the molecule is slowed down. The image was featured on the cover of the March issue of Nature Methods.